Humanitarian Aid in the Age of AI
In 2024, nearly 300 million people (almost 4% of the population) need humanitarian assistance and protection. 1 out of 5 children is living in or fleeing from conflict. 1 in 73 people is forcibly displaced.
With rising stats like these, the humanitarian sector is facing a dire situation that requires innovative solutions, and artificial intelligence (AI) can help.
AI is capable of performing tasks such as learning, comprehension, problem solving, decision making, creativity and autonomy that would have previously required human brainpower. In the humanitarian context, this enhanced efficiency and advanced capability can help organisations identify and prioritise areas of need and target resources more effectively.
AI is being used to both prevent and react to humanitarian crises, and its potential to reshape the humanitarian sector is profound.
How AI can help
AI offers innovative solutions to some of the most pressing challenges faced by humanitarian organisations – from preventing disasters in the first place to distributing aid to disaster-affected people.
Disaster Risk Management
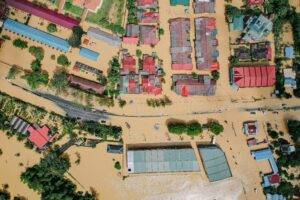
AI is being used to make predictions for natural disasters, such as floods and earthquakes.
AI is changing the way disaster risk is modelled and managed. Researchers are using machine learning in the field of Disaster Risk Management (DRM) to find patterns in given data and make predictions. They do so by analysing vast datasets, such as satellite imagery, seismic data, and weather patterns, to predict events like hurricanes, earthquakes, or droughts. These predictions enable governments and humanitarian organisations to issue early warnings and prepare communities.
For example, Google’s FloodHub is one of many models that provide real-time flood predictions, helping to mitigate the devastating effects of flooding in vulnerable regions. The end-to-end flood warning system is designed to forecast future river stages and flood inundation, whereafter alerts are distributed to government authorities, emergency response agencies, and the affected population.
Analysis and response
AI systems can also be used to assist humanitarian responses. The World Food Programme partnered with Google Research to set up the SKAI platform to automatically assess damage after disasters and speed up humanitarian response. SKAI’s machine learning model detects damage by comparing imagery of buildings before and after a disaster. This reduces the workload on human analysis and cuts humanitarian response times from weeks down to days, getting help to disaster areas much more efficiently.
Communications and connection
In the aftermath of a crisis, AI can facilitate engagement between humanitarians and affected communities. Numerous organisations have implemented AI-powered chatbots to connect crisis-affected communities, refugees and migrants with real-time information, educational resources and disaster alerts.
UNICEF’s Aurora chatbot, for example, provides information to migrants travelling through the Darien jungle and Central America. The bot makes it easy to access vetted information on humanitarian aid available along the route, self-care and safety recommendations, and news, so migrants can make informed decisions.
And to help refugees locate missing family members, the International Committee of the Red Cross developed the Trace the Face tool. It leverages facial recognition technology to automate searching and matching photos, making the process more efficient and effective.
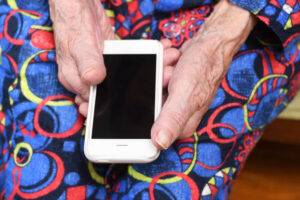
AI-powered chatbots connect migrants and refugees with vital information.
How AI can harm
While there is vast potential for AI to help disaster or crises-affected people and facilitate humanitarian action, this fallible technology also has the potential to do harm to groups who are already vulnerable. These are some of the concerns humanitarians need to keep top of mind when engaging with AI.
Limited access
Practically, deploying AI systems such as chatbots in areas struggling with digital access can be problematic. Humanitarian crises or disasters could make it even more difficult than usual to access digital services and the internet.
Flawed data leads to flawed outcomes
AI is also only as good as the data that feeds it. These systems benefit from robust datasets and infrastructure, which may be harder to capture in conflict-affected areas or historically underserved communities. If the data is out of date or incorrect, the system may fail to account for changing variables like human behaviour and environmental shifts, leading to incomplete or inaccurate predictions. Models could also reinforce errors, inequalities, and historical biases that were introduced with flawed data.
Human biases
AI for humanitarian action: Human rights and ethics explains how biases and other flaws in data can infect a system at different stages. It starts with the developers themselves. Their conscious or unconscious biases can be coded into a model. Certain machine learning systems have shown racial or gender biases. For example, a machine learning tool used by Amazon to review job applications disproportionately rejected women, and facial recognition tech has been worse at recognising non-white faces.
Consent and privacy
To gather the data on humans needed for machine learning, you need their consent. But, in the humanitarian context, consent can be difficult to establish. Due to the inherent power imbalance between humanitarian organisations and beneficiaries, it may not be freely given. Beneficiaries may fear that a refusal to consent to the use of data would bar them from accessing aid.
The data collected could also include sensitive information about refugees, disaster victims and vulnerable populations. Once gathered, the data could be misused or stolen, exposing individuals to risks like identity theft, discrimination, or surveillance.
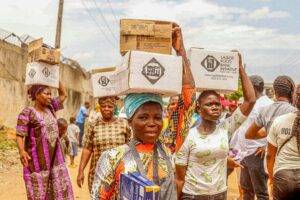
The use of AI in humanitarian aid should prioritise meeting the needs of vulnerable populations.
Defining the standard
AI gains more traction by the minute. There is an urgent need to ensure that AI does not infringe on human rights, especially when it is used for humanitarian, development and peace operations. This requires the development of a universally agreed upon framework that is bound by law – a mammoth task with its own set of challenges.
Just as we need a localised approach to general humanitarian aid, we need a localised approach to the implementation of AI systems. Building inclusive AI requires collaboration with affected communities to ensure tools address local needs and cultural contexts.
It is up to those working in humanitarian aid to ensure responsible, context-sensitive implementation of AI that prioritises the needs of the most vulnerable populations.